How AI is Revolutionizing APT Detection and Prevention | The Future of Cybersecurity
Advanced Persistent Threats (APTs) pose a significant cybersecurity challenge, using stealthy techniques to infiltrate networks and exfiltrate data over long periods. Traditional security measures struggle to detect APTs, but Artificial Intelligence (AI) is revolutionizing cybersecurity with machine learning, behavioral analysis, and automation. AI-powered tools analyze large datasets, detect anomalies, and predict cyber threats in real-time, improving incident response and minimizing damage. AI enhances deception techniques, automates threat hunting, and helps mitigate zero-day attacks, but challenges like adversarial AI and false positives persist. As AI advances, integrating it with zero-trust security and federated learning will be crucial in combating APTs effectively.
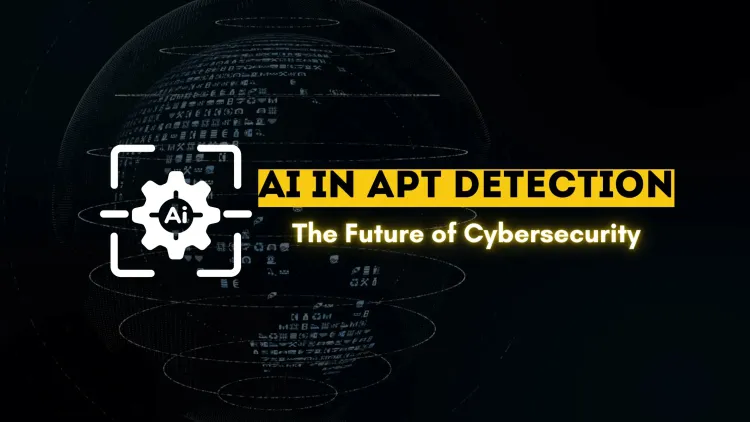
Table of Contents
- Introduction
- Understanding Advanced Persistent Threats (APTs)
- How AI Helps Detect and Stop APTs
- Challenges of Using AI in APT Detection
- The Future of AI in Combating APTs
- Conclusion
- Frequently Asked Questions (FAQ)
Introduction
Advanced Persistent Threats (APTs) are among the most dangerous and sophisticated cyber threats, targeting organizations, governments, and critical infrastructure. These stealthy attacks are carried out by well-funded adversaries who use advanced techniques to infiltrate networks, steal sensitive data, and maintain long-term access. Traditional security measures often struggle to detect and mitigate APTs due to their complexity and persistence.
With the rise of Artificial Intelligence (AI) in cybersecurity, organizations are exploring AI-driven solutions to detect and stop APTs more effectively. In this blog, we will discuss how AI can help in identifying and mitigating APTs, the benefits it brings, and the challenges it faces.
Understanding Advanced Persistent Threats (APTs)
APTs are cyberattacks conducted by highly skilled adversaries with a strategic objective. These attacks typically follow a multi-stage process:
- Reconnaissance – Attackers gather intelligence on the target.
- Initial Compromise – Exploiting vulnerabilities, phishing, or social engineering to gain access.
- Establishing Foothold – Deploying malware to maintain access.
- Lateral Movement – Expanding control within the network.
- Data Exfiltration – Stealing sensitive information.
- Maintaining Persistence – Ensuring continued access to the compromised system.
APTs often bypass traditional security defenses by leveraging zero-day vulnerabilities, stolen credentials, and sophisticated evasion techniques.
How AI Helps Detect and Stop APTs
AI-driven cybersecurity tools leverage machine learning (ML), deep learning, and behavioral analytics to detect anomalies, predict attacks, and automate threat response. Here’s how AI can assist in combating APTs:
1. Behavioral Analysis for Anomaly Detection
Traditional security solutions rely on rule-based detection, which struggles against unknown threats. AI uses behavioral analytics to establish a baseline of normal network activity and detect anomalies that may indicate an APT attack.
- Example: AI can identify unusual login patterns, unexpected data transfers, or unauthorized access attempts.
2. Threat Intelligence and Predictive Analytics
AI can process vast amounts of cybersecurity data, including threat intelligence feeds, dark web monitoring, and attack patterns, to predict potential threats before they materialize.
- Example: AI-powered Security Information and Event Management (SIEM) systems can correlate threat indicators across multiple data sources in real time.
3. Automated Incident Response and Threat Hunting
AI-powered tools can automate threat hunting, identifying potential APT indicators and responding instantly to minimize damage.
- Example: AI-driven Security Orchestration, Automation, and Response (SOAR) systems can isolate compromised systems, block malicious IPs, and alert security teams.
4. AI-Driven Deception Techniques
AI enhances deception technologies such as honeypots and decoy systems to mislead attackers and collect intelligence on their tactics.
- Example: AI-powered honeynets dynamically adapt to adversarial behavior, tricking APT actors into revealing their techniques.
5. Detecting Fileless and Polymorphic Malware
APTs often use fileless malware and advanced obfuscation techniques. AI can detect these threats by analyzing system behavior rather than relying on signatures.
- Example: AI-based endpoint detection and response (EDR) solutions track abnormal process execution and memory manipulations.
Challenges of Using AI in APT Detection
While AI offers significant advantages in APT detection and mitigation, there are challenges that need to be addressed:
- False Positives – AI models may generate high false-positive rates, requiring continuous refinement to improve accuracy.
- Adversarial Attacks – Attackers can manipulate AI models through adversarial machine learning, making AI less effective.
- Resource Intensive – AI-powered cybersecurity solutions require substantial computational resources and skilled personnel to manage them.
- Data Privacy Concerns – AI-driven security solutions need access to large datasets, raising privacy and compliance issues.
The Future of AI in Combating APTs
As AI technology advances, its role in cybersecurity will become more prominent. Future developments may include:
- Federated Learning for Secure AI Models – Enhancing AI security without exposing sensitive data.
- Quantum AI for Cryptographic Security – Using quantum computing to strengthen AI-driven malware detection.
- AI and Zero Trust Security – Integrating AI with zero trust architectures to verify and monitor all network activity continuously.
- Self-Learning AI Systems – AI models that adapt to new attack techniques without human intervention.
Conclusion
AI is revolutionizing the way organizations detect and respond to APTs. By leveraging machine learning, behavioral analytics, and automation, AI-powered cybersecurity tools enhance threat detection, response, and mitigation efforts. However, AI is not a silver bullet; it must be combined with strong security policies, skilled analysts, and continuous monitoring to be truly effective.
As cyber threats continue to evolve, investing in AI-driven security solutions will be crucial in staying ahead of sophisticated adversaries and protecting critical infrastructure from APT attacks.
Frequently Asked Questions (FAQ)
How does AI detect Advanced Persistent Threats (APTs)?
AI detects APTs by analyzing behavioral patterns, detecting anomalies, and leveraging machine learning models to identify malicious activities in real time.
Can AI prevent zero-day attacks?
Yes, AI uses predictive analytics and behavioral analysis to detect and mitigate zero-day attacks before they can cause significant damage.
What role does machine learning play in APT detection?
Machine learning models analyze vast datasets of attack patterns, detect deviations from normal behavior, and classify potential threats accurately.
How does AI improve threat hunting?
AI automates threat hunting by continuously scanning network activity, correlating data from multiple sources, and identifying hidden threats.
Can AI differentiate between normal and malicious activity?
Yes, AI models learn baseline behavior patterns and detect anomalies that deviate from normal user and system activity.
What are AI-driven honeypots, and how do they work?
AI-driven honeypots are decoy systems designed to attract and analyze attacker behavior, providing valuable threat intelligence.
How does AI contribute to cybersecurity automation?
AI automates threat detection, incident response, and security orchestration, reducing the workload for security analysts and improving response times.
Can AI be used for proactive cybersecurity defense?
Yes, AI proactively identifies vulnerabilities, monitors attack trends, and predicts potential threats before they materialize.
What is adversarial AI, and how does it impact cybersecurity?
Adversarial AI involves cybercriminals manipulating AI models to bypass security measures, making AI security a continuous challenge.
How does AI help in identifying insider threats?
AI analyzes employee behavior, access patterns, and unusual activity to detect potential insider threats before they cause harm.
Is AI effective against polymorphic and fileless malware?
Yes, AI detects these advanced threats by analyzing execution behaviors and identifying suspicious patterns rather than relying on signatures.
How does AI integrate with SIEM systems?
AI enhances SIEM (Security Information and Event Management) by automating threat detection, correlating logs, and reducing false positives.
Can AI replace human security analysts?
No, AI augments human analysts by automating repetitive tasks and improving detection accuracy, but human expertise remains essential.
What are the benefits of AI-driven endpoint detection and response (EDR)?
AI-powered EDR solutions detect anomalies, block threats in real-time, and provide forensic analysis to investigate cyber incidents.
How does AI enhance network security?
AI continuously monitors network traffic, identifies anomalies, and blocks malicious activities before they escalate.
Can AI stop ransomware attacks?
Yes, AI detects early signs of ransomware encryption and blocks malicious processes before data is compromised.
What is AI-driven deception technology?
AI-powered deception techniques use fake assets, dynamic honeypots, and false information to mislead attackers and gather intelligence.
How does AI improve firewall security?
AI-enhanced firewalls use machine learning to analyze network traffic, detect anomalies, and prevent cyber threats more efficiently.
Can AI detect threats in encrypted traffic?
Yes, AI can identify malicious behavior in encrypted traffic by analyzing metadata and usage patterns without decryption.
How does federated learning improve AI-based cybersecurity?
Federated learning enables AI models to learn from distributed data sources without exposing sensitive information, enhancing security and privacy.
What are the limitations of AI in APT detection?
Challenges include false positives, adversarial AI attacks, high resource requirements, and the need for continuous model training.
How does AI contribute to zero-trust security?
AI enhances zero-trust security by continuously verifying users, monitoring access behavior, and detecting anomalies in real time.
What role does AI play in dark web monitoring?
AI scans the dark web for leaked credentials, stolen data, and potential cyber threats, providing early warning signs of breaches.
How does AI detect social engineering attacks?
AI analyzes email content, communication patterns, and behavioral cues to identify phishing attempts and social engineering scams.
Can AI prevent credential stuffing attacks?
Yes, AI detects abnormal login attempts, analyzes IP addresses, and blocks automated credential stuffing attacks.
How does AI help in cybersecurity compliance?
AI automates compliance monitoring, detects policy violations, and ensures organizations meet regulatory cybersecurity standards.
What is the impact of quantum computing on AI-driven cybersecurity?
Quantum computing can enhance AI models for cybersecurity but also poses a risk by breaking traditional encryption methods.
How does AI handle real-time cybersecurity alerts?
AI prioritizes security alerts, reduces noise from false positives, and provides actionable insights for rapid incident response.
What is self-learning AI in cybersecurity?
Self-learning AI adapts to new attack techniques, continuously improves threat detection models, and reduces reliance on human intervention.
Can AI detect threats in cloud environments?
Yes, AI monitors cloud traffic, detects unauthorized access, and prevents cloud-specific attacks like misconfigurations and API exploits.