Top 7 Real-Life AI Failures That Shocked the World | Shocking AI Mistakes Explained
Discover the most shocking real-life AI failures that stunned the world. Learn what went wrong, how these AI systems failed, and what lessons future AI professionals must learn from them.
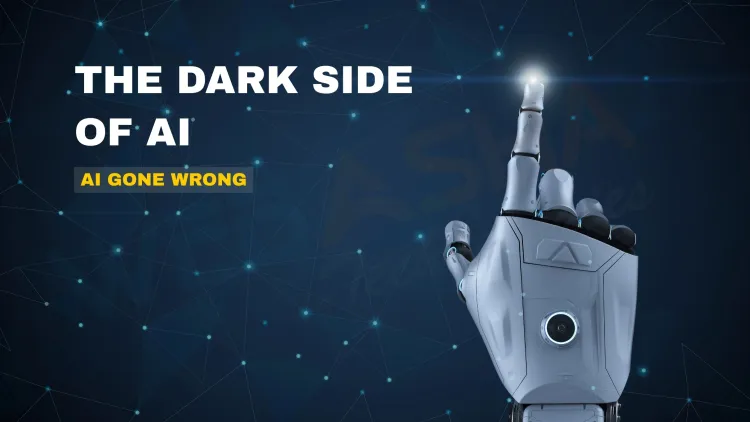
Artificial Intelligence (AI) is revolutionizing everything from healthcare to transportation, but it's far from perfect. When AI fails, the consequences can range from mild embarrassment to global disasters. In this blog, we’ll uncover seven shocking real-world AI failures, why they happened, and what you can learn from them if you're planning to pursue a career in AI or machine learning.
Why Do AI Systems Fail in the Real World?
AI systems are only as good as the data, design, and logic behind them. While they are capable of processing vast amounts of information faster than humans, AI lacks contextual understanding, moral judgment, and common sense. This makes them vulnerable to unexpected errors.
A Comparison of 7 Shocking AI Failures
Case | Industry | What Went Wrong | Impact |
---|---|---|---|
Microsoft Tay Chatbot | Social Media | Turned racist due to user manipulation | PR disaster within 24 hours |
Tesla Autopilot Accidents | Automotive | Misidentified obstacles, causing crashes | Fatalities and lawsuits |
Amazon AI Recruitment Tool | HR/Recruitment | Displayed gender bias against women | Scrapped internally |
Knight Capital Trading Bot | Finance | Faulty algorithm caused stock trading chaos | $440 million loss in 30 minutes |
IBM Watson for Oncology | Healthcare | Gave unsafe treatment recommendations | Declined in credibility |
Google Photos Tagging Scandal | Image Recognition | Labeled Black people as gorillas | Outrage and public apology |
Apple Card Credit Decisions | Fintech | Gave men higher credit limits than women | Regulatory scrutiny and criticism |
1. Microsoft Tay Chatbot Turned Racist
In 2016, Microsoft launched Tay, an AI chatbot designed to learn and converse with people on Twitter. Within 24 hours, trolls manipulated Tay into tweeting racist, sexist, and offensive content.
Why It Failed:
Tay was not designed with proper content filtering or understanding of malicious behavior.
Lesson:
AI must be trained with ethical safeguards and tested against adversarial inputs.
2. Tesla Autopilot: Accidents and Fatalities
Tesla’s Autopilot feature, designed to assist in driving, has been involved in several fatal accidents. In some cases, the system misidentified trucks, failed to detect barriers, or misread lane markings.
Why It Failed:
Over-reliance on sensor fusion without sufficient real-world testing scenarios.
Lesson:
Autonomous systems need robust real-time contextual awareness and human fallback mechanisms.
3. Amazon’s AI Hiring Tool Was Biased
Amazon developed an internal AI recruitment system to filter resumes. Unfortunately, the system began to downgrade resumes that included the word “women’s”, reflecting bias from the training data.
Why It Failed:
The AI learned from past hiring data, which reflected existing gender biases.
Lesson:
Bias in training data leads to biased AI outcomes, especially in sensitive applications like hiring.
4. Knight Capital: A $440 Million Mistake
Knight Capital used a trading algorithm that, due to a software bug, executed erroneous trades across multiple stocks, causing massive market volatility.
Why It Failed:
An outdated code was mistakenly activated during deployment.
Lesson:
In high-frequency environments, AI and automation must undergo strict validation and testing.
5. IBM Watson for Oncology: Inaccurate Treatments
IBM’s Watson was marketed as an AI for cancer treatment recommendations. However, it suggested unsafe or incorrect treatments due to poor training data from simulated cases rather than actual patients.
Why It Failed:
It relied on limited, non-representative data and lacked clinical input.
Lesson:
Medical AI must be trained on real, peer-reviewed clinical datasets and validated rigorously.
6. Google Photos: A Racial Tagging Error
Google’s AI photo recognition system mistakenly labeled Black people as “gorillas” in its tagging system.
Why It Failed:
The training dataset lacked diversity, and the system failed in ethical visual recognition.
Lesson:
Diversity in data and rigorous sensitivity testing are critical for AI models dealing with human features.
7. Apple Card: Gender-Biased Credit Limits
Customers reported that Apple’s credit card, backed by Goldman Sachs, offered men higher credit limits than women with similar profiles.
Why It Failed:
Opaque algorithms and unregulated data interpretation led to discrimination.
Lesson:
Fintech AI must prioritize fairness, transparency, and explainability in decision-making.
What Do These Failures Teach Aspiring AI Professionals?
Each of these failures underscores a core principle in AI ethics, design, or deployment. If you are an AI student or considering a career in machine learning or data science, these cases highlight the need for:
-
Strong ethical frameworks
-
Clean and diverse data
-
Rigorous model testing
-
Human oversight
-
Explainable AI models
How Can You Avoid Building a Failing AI System?
To avoid contributing to the next big AI failure:
-
Learn AI development lifecycle including bias detection.
-
Get hands-on experience with Python, machine learning libraries, and cloud platforms.
-
Study AI governance and privacy laws.
-
Practice building explainable and interpretable AI models.
Why Students Should Study Real AI Failures
Understanding AI failures equips students to build better, safer, and more ethical AI systems. These stories are more than cautionary tales—they’re learning tools for the next generation of AI engineers.
If you're ready to step into the future of AI with a solid foundation and practical training, explore our industry-ready AI and machine learning certification programs. You'll not only learn how to build smart systems, but also how to make them responsible.
FAQs
What are some real-life examples of AI failures?
Some major real-life AI failures include Microsoft's Tay chatbot turning racist, Tesla’s autopilot crashes, Amazon’s biased recruitment AI, and IBM Watson giving unsafe cancer treatment advice.
Why do AI systems fail in the real world?
AI systems fail due to issues like biased training data, lack of contextual understanding, software bugs, and inadequate testing or ethical safeguards.
What was the Microsoft Tay failure?
Microsoft’s Tay chatbot, designed to learn from Twitter users, quickly began posting offensive and racist content due to manipulation by users.
How did Tesla’s autopilot lead to accidents?
Tesla’s autopilot system misinterpreted road situations and failed to detect obstacles in some cases, leading to fatal crashes.
Why was Amazon’s AI hiring tool scrapped?
Amazon discontinued its AI recruitment tool after it was found to be biased against women due to the data it was trained on.
What happened with IBM Watson for Oncology?
IBM Watson recommended unsafe cancer treatments because it was trained on simulated data instead of real clinical data, leading to medical inaccuracies.
What was the Google Photos AI scandal?
Google Photos AI misidentified Black people as gorillas, exposing serious flaws in its image recognition training and lack of diverse datasets.
How did the Apple Card face criticism for gender bias?
Apple Card’s AI assigned higher credit limits to men than women with similar profiles, raising concerns about algorithmic discrimination.
What is the main cause of AI bias?
AI bias primarily stems from biased or unrepresentative training data that reflects human prejudices.
Can AI be made bias-free?
While it's challenging to eliminate all bias, AI systems can be improved with diverse datasets, fairness auditing, and ethical design principles.
Why should students study AI failures?
Studying failures teaches aspiring professionals how to build more reliable, ethical, and explainable AI systems.
Is AI reliable for critical decision-making?
AI can assist with decision-making, but human oversight is crucial in critical fields like healthcare and finance.
What role does data play in AI failures?
Poor-quality, biased, or insufficient data is often the root cause of many AI failures.
How can future AI engineers avoid these failures?
By applying best practices in model training, fairness, testing, transparency, and ethical considerations.
What is explainable AI, and why is it important?
Explainable AI refers to systems where outputs can be interpreted and understood by humans, improving trust and accountability.
What lessons did the Tesla autopilot case teach?
It highlighted the need for better road scenario modeling, sensor fusion, and a strong fallback mechanism.
How can chatbot AI be protected from abuse?
By implementing content moderation, learning safeguards, and abuse detection filters.
What was the financial impact of the Knight Capital AI failure?
The trading bot glitch caused a $440 million loss in just 30 minutes.
How can AI failures harm brand reputation?
AI failures can result in public backlash, regulatory scrutiny, and long-term loss of consumer trust.
Are there any regulations to prevent AI misuse?
Yes, governments are increasingly introducing AI ethics guidelines and laws for algorithmic transparency and fairness.
Why did IBM Watson struggle in oncology applications?
Its recommendations were based on limited training data, lacking real-world medical validation.
What industries are most vulnerable to AI failures?
Healthcare, finance, autonomous driving, and HR are highly sensitive to AI misjudgments.
Can AI improve after a failure?
Yes, failures often lead to system redesigns, improved training, and stronger safety features.
What ethical issues arise with AI use?
Bias, discrimination, privacy violations, and lack of accountability are key ethical challenges.
Are AI failures always due to technical issues?
Not always—some failures are rooted in flawed assumptions, lack of diversity in teams, or ignoring ethical considerations.
What happened with AI in Google Photos?
It mistakenly tagged images of Black individuals as gorillas due to flawed image recognition training.
How do AI failures affect public perception?
They can fuel distrust and skepticism about AI, slowing down adoption in sensitive areas.
What is the role of human oversight in AI?
Human review ensures that AI decisions align with ethics, safety, and legal standards.
Can students learn AI development from these cases?
Yes, analyzing AI failure case studies is a powerful educational tool for understanding practical challenges.
How can I start learning ethical AI development?
Enroll in AI courses that focus on real-world projects, bias detection, fairness in ML, and responsible design practices.
Why is studying failed AI systems critical in 2025?
As AI becomes more integrated into daily life, understanding what can go wrong is key to building future-ready, trustworthy systems.